In the rapidly evolving landscape of data management and analytics, the role of the data engineer has undergone a significant transformation. Traditionally, data engineers were tasked with building and managing data pipelines, helping ensure data quality, and supporting the integration of analytics into business processes. However, the demands of modern data ecosystems have expanded the scope of data engineering to include advanced responsibilities such as cloud infrastructure management, real-time data processing, cost and multi-cloud optimization. This blog explores the key differences between traditional and modern data engineers, the challenges they face, and the skills required to help Data Engineers succeed in today’s AI driven world.
Traditional Data Engineering
Traditionally, Data Engineers were tasked with transforming raw data into a usable form that meets the needs of data consumers, including data scientists, analysts, and business users. It focused on the timely delivery of data; ensuring it is properly cataloged, governed, and compliant with relevant regulations.
Data Engineers play a crucial role in the success of data and analytics (D&A) initiatives. Without them, organizations faced increased costs, deployment delays, and challenges related to data integration, quality, and availability.
Key Responsibilities
- Building and Managing Data Pipelines: Traditional data engineers are responsible for the creation and maintenance of data pipelines, ensuring that data flows efficiently from source systems to data warehouses and/or data lakes.
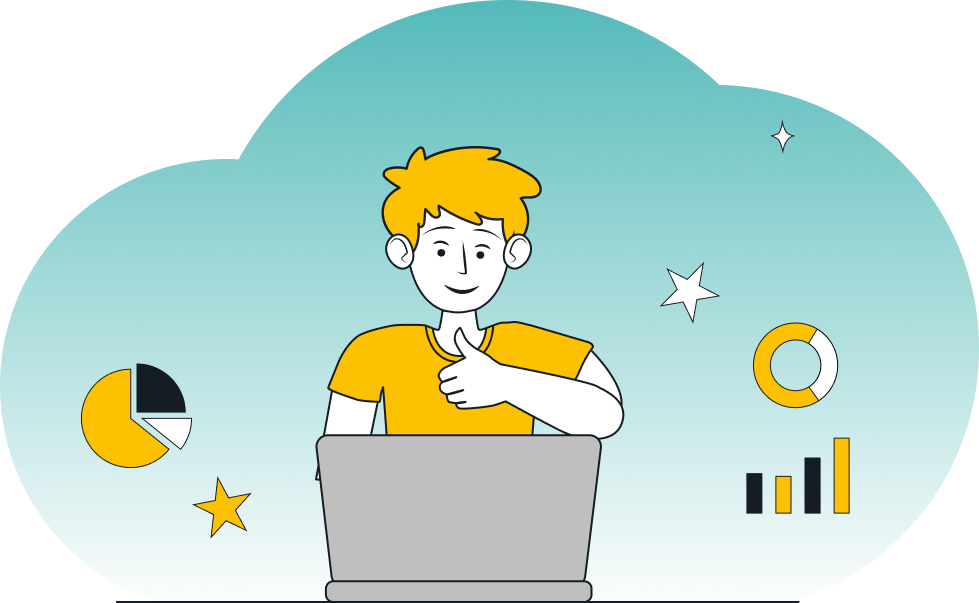
- Operationalizing Data Delivery: Data Engineers ensure that data delivery is automated and operationalized for production-level deployments, minimizing manual intervention.
- Curating Datasets: They support non-technical users, such as data scientists, by curating datasets and providing the necessary infrastructure for self-service data preparation.
- Supporting Analytics Deployments: Traditional data engineers help deploy analytics and data science outputs into business processes and applications, ensuring they are integrated seamlessly.
Skills Required
- Working with Diverse Datasets: Traditional data engineers must be adept at parsing and understanding various data formats.
- Collaboration with Domain Experts: They work closely with data scientists, analysts, and domain experts to ensure data is properly managed.
- Data Governance and Security: Ensuring compliance with data governance and security policies is a critical aspect of their role.
Modern Data Engineering
With the ongoing popularity of Generative AI, Large Language Models (LLMs), cloud native data warehouses or platforms like Snowflake, Databricks, AWS Redshift, Google Big Query, etc the expectations of data engineers have evolved.
Modern data engineers are often responsible for designing, building, and maintaining the infrastructure necessary for collecting, storing, and processing large volumes of data. They work closely with data scientists, analysts, and other stakeholders to ensure that data systems are robust, efficient, cost optimized and reliable.
Additionally, data engineers are expected to be data architects, understand the cloud native platforms, the cloud service provider implications, pricing models to optimize consumption and much more.
i.e., they are expected to have skills of Distinguished Data Engineers, of whom there are too few in existence. If we adopted a term from the venture community, these data engineers would be labeled as “Decacorns”.
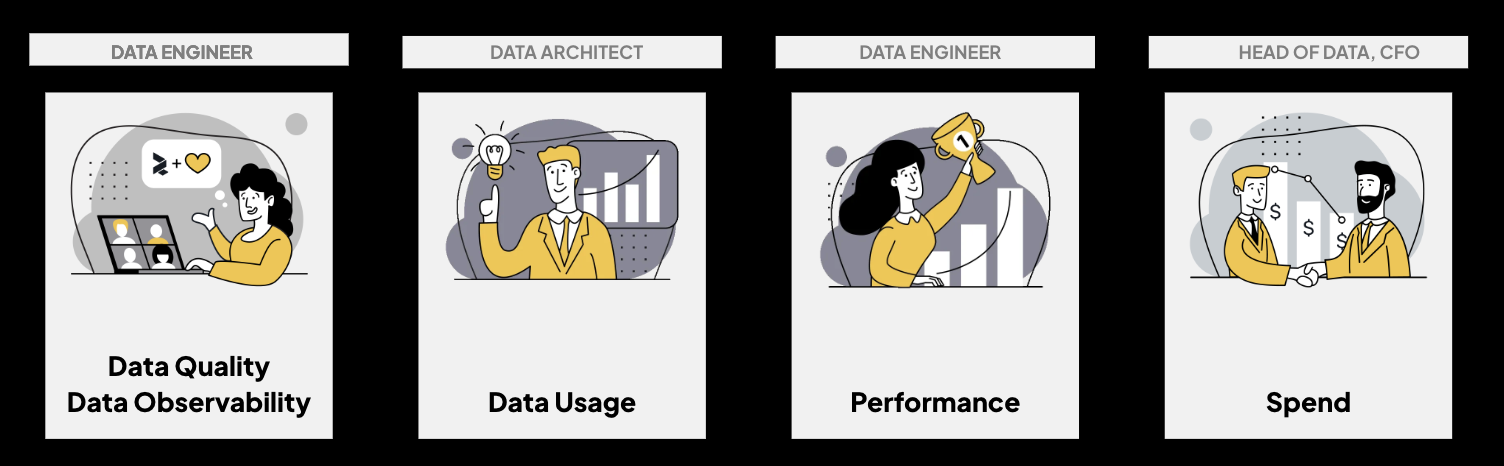
Key Expectations
- Data Pipeline Development: Modern data engineers design, develop, and maintain scalable data pipelines for the efficient collection, transformation, and loading (ETL) of data from various sources.
- Database Management: They administer and optimize relational and NoSQL databases to ensure high performance and availability
- Data Warehousing: Modern data engineers build and manage data warehouses that store both structured and unstructured data, ensuring data is organized and accessible.
- Spend Optimization: A critical aspect of the modern data engineer’s role is ensuring that data usage and associated costs are optimized, preventing licensing and spending overruns.
- Data Integration: These engineers integrate data from various sources, including APIs, third-party services, and internal databases, ensuring consistency and accuracy.
- Data Quality and Governance: Modern data engineers implement data quality checks and governance policies to maintain data integrity, security, and compliance.
- Collaboration: They work closely with data scientists, analysts, and business stakeholders to understand data needs and provide the necessary infrastructure and tools.
- Performance Optimization: Modern data engineers are experts in troubleshooting and optimizing data systems for performance, reliability, and scalability.
- Multi-Cloud Expertise: Knowledge of popular cloud platforms and their interworking is essential for modern data engineers.
- Documentation: They document data processes, architecture, and infrastructure to ensure transparency and maintainability.
Challenges Faced by Modern Data Engineers
The expanded role of the modern data engineer comes with its own set of challenges:
- Managing Complex Data Ecosystems: With the proliferation of data sources and the need for real-time processing, modern data engineers must manage increasingly complex data ecosystems.
- Ensuring Data Quality at Scale: As data volumes grow, maintaining data quality and governance becomes more challenging, requiring advanced tools and techniques.
- Balancing Performance and Cost: Modern data engineers must optimize data systems for performance while keeping costs under control, especially in cloud environments.
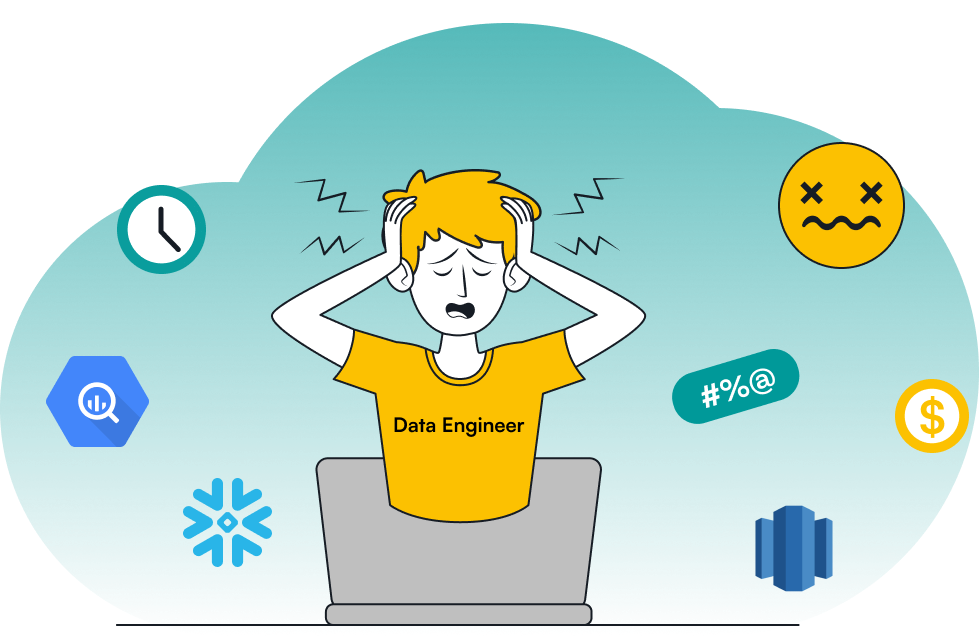
- Keeping Up with Technological Advancements: The rapid pace of innovation in data technologies means that modern data engineers must continuously update their skills and knowledge.
- Collaboration Across Teams: Modern data engineers must work closely with various stakeholders, including data scientists, analysts, and business users, to ensure that data systems meet organizational needs.
- Fire-fighting: While data engineers need to build data products, pipelines, ensure quality, they are invariably involved in routine and preventable operational and tactical tasks impacting outcomes.
We Must Assist Modern Data Engineers
To support modern data engineers in their expanded roles, organizations need to invest in the following areas:
- Proper Tooling: Modern data engineers should have access to the latest tools for data integration, quality assurance, and performance optimization is crucial.
- Continuous Learning and Development: Organizations should invest in the ongoing education and skill development of their data engineers to keep them up-to-date with the latest technologies.
- Collaboration Platforms: Implementing collaboration platforms that facilitate communication between data engineers, data scientists, and business stakeholders can help streamline processes.
- Scalable Infrastructure: Modern data engineers need access to scalable cloud infrastructure that can handle large volumes of data and complex processing requirements.
- Automation: Automating routine tasks, such as data pipeline monitoring and maintenance, can free up modern data engineers to focus on more strategic initiatives.
- Generative AI: While still early, adoption of AI can help augment data engineers.
Conclusion
The role of the data engineer has evolved significantly, moving from a traditional focus on data pipeline management to a modern emphasis on scalable infrastructure, cloud optimization, spend management and real-time data processing. As organizations continue to rely on data-driven decision-making and explore the adoption of AI, the importance of modern data engineers will only grow. To address the challenges faced by today’s data engineers, organizations must ensure that they have the necessary tools and resources to support their data initiatives and drive business success.